One of the biggest incentives in academia is proof of the impact of your research. This often helps with promotion and tenure. Whilst measures like the impact factor have been repeatedly demonstrated to be compromisable, citations are still recognised by researchers as the gold standard for academic impact.
With citations of non-traditional research outputs (NTROs) such a datasets, there are a few things to consider:
- Citations are rarely in the reference list
- A lot of usage is self citation, which can be either in 1 paper, or many
As such, we are thinking hard about tracking impact for researchers to reward them for their good practice.
Altmetrics are metrics and qualitative data that are complementary to traditional, citation-based metrics. You can learn more about them here. We believe that by tracking both Altmetric scores and citation counts, we can better understand re-use of NTROs to help better incentivise open scholarly practices.
Recently we added a way to sort outputs based on their citation count or Altmetric score. This works for all repositories powered by Figshare and is new in the repository space. This allowed us to easily dig through re-used content to see the types that are having success.

When we begin to scratch the surface, we see several ‘types’ of dataset that get lots of citations, such as ‘databases’. eg.
Face Research Lab London Set
https://doi.org/10.6084/m9.figshare.5047666.v5
Global Aridity Index and Potential Evapotranspiration (ET0) Climate Database v2
https://doi.org/10.6084/m9.figshare.7504448.v3
Data from: The Global Avian Invasions Atlas – A database of alien bird distributions worldwide
https://doi.org/10.6084/m9.figshare.4234850.v1
We also see well described standalone datasets having impact. Such as,
Journal subscription costs – FOIs to UK universities. https://doi.org/10.6084/m9.figshare.1186832.v23
It seems the commonality between those listed above is that they do not necessarily need to be associated with a paper. They are the output of the research, and as such have been well described in the metadata.
The past 12 months have been unique in the scholarly publishing landscape, in that there was so much focus on one topic, COVID-19. As expected, this new rapid publishing drive and a large audience of interested parties has led to large citation counts. E.g.:
“COVID-19 severity correlates with airway epithelium-immune cell interactions identified by single-cell analysis” https://doi.org/10.6084/m9.figshare.12436517.v2
So are there any datasets that are published to support a publication and then re-used by other research groups?
If we take 2 datasets with a relatively high number of citations, that reference a paper in their metadata and compare them, we can see a key difference. The datasets we are considering are:
Green algal transcriptomes for phylogenetics and comparative genomics
https://doi.org/10.6084/m9.figshare.1604778.v1
And
EEG correlates of social interaction at distance
https://doi.org/10.6084/m9.figshare.1466876.v8

As can be seen in the above table, whilst there are no huge differences in the metrics that are listed on the landing page, using citation counts as a proxy for re-use highlight that one dataset has largely been re-used by the same authors as the dataset. The other is largely re-used by a distinct set of authors. Of note, the only re-use by non authors for the dataset titled ‘EEG correlates of social interaction at distance’ is a paper whose sole function is a re-analysis of the dataset – Electrocortical correlations between pairs of isolated people: A reanalysis.
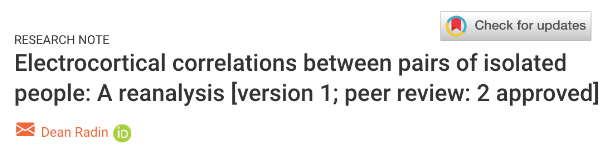
In summary, there are a few things that help. Primarily, Metadata – and lots of it, a readme too! Both examples of re-use investigated above have a ‘readme’ file or an ‘instructions’ text file. Both of these datasets authors have therefore asked the question – Does this have enough metadata to be re-used as a single output?
For the future, should we be recognising re-use by other authors to avoid datasets being cited by their authors in all their papers, even if not used? The company Scite is focussed on smart citations for better research, initially focussed on retractions and understanding the sentiment of citations. This seems to be a huge task for citing datasets going forward, to understand the ‘why’ of each citation, in order to elucidate real meaning in citation counts.
If you have any ideas of what level of transparency we should be focussing our efforts on here, or if you would like to explore this theme further, we’d love to hear from you at info@figshare.com